How will the COVID19 spread? How many people will be infected and how many fatalities will we face? Answering these questions requires making data driven predictions about the future state of the spread of the virus. This is not an easy task.
I found out first hand when helping a local hospital to make some predictions on their required IC capacity. Not being an epidemiologist, I replied that I wasn’t equipped to help make actual predictions. I could, however, provide some insight into the possible effects of these predictions on their local IC capacity. Over the course of this project, I learned two things that make a datadriven forecast the spread of Covid19 so difficult to generate.
Complexity of modeling
Modeling the spread of a virus is complex. Transmission dynamics, reflecting how a disease spreads, requires measures of contagiousness, length of incubation time, duration of how long a patient is infectious to name a few. Clinical dynamics, which describe the effects on clinical practice, require the fatality rate, hospitalization rate, length of the hospital stay, to name a few [1]. On top of that, there are policy interventions such as a lockdown or social distancing which (hopefully) change the course of the spread of the virus.
So modeling the spread is not as straightforward as extrapolating historic results into the future, it requires an understanding of the mathematical models as well as the dynamics of a clinical operation and impact of policy. Not an easy task.
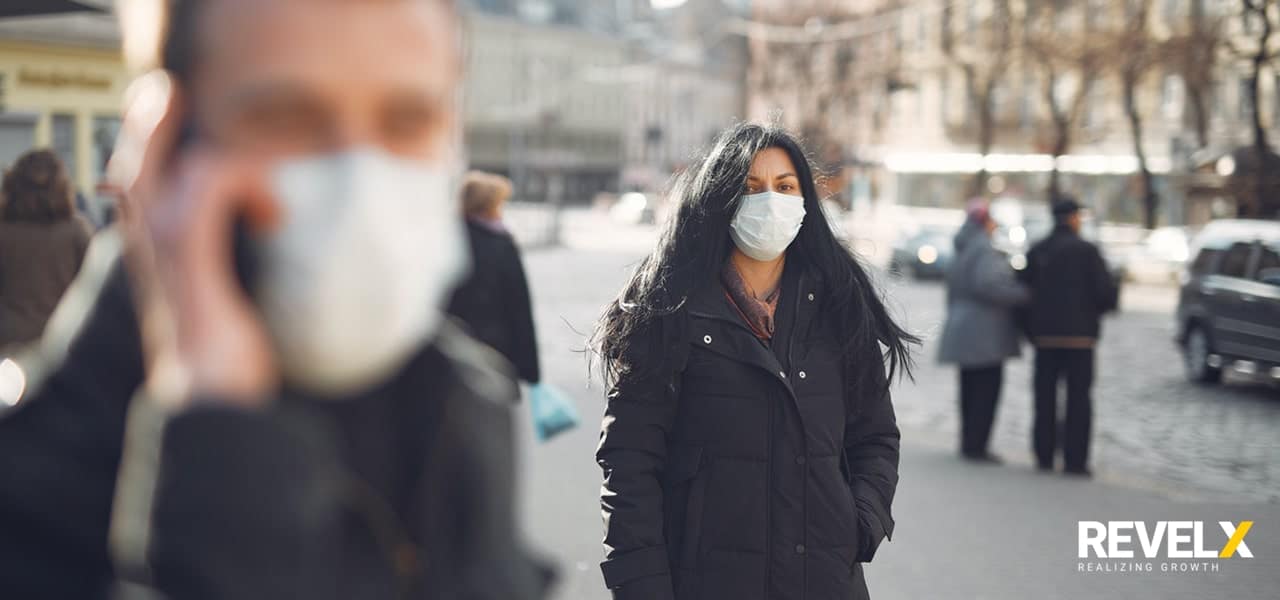
The actual data in “data driven”
To make any type of data-driven prediction, the quality of the data matters, a lot. In the case of COVID-19, this is not as clear cut as it may appear on the surface. Due to limited testing capability, most countries have no actual account of how many people have been infected with the virus, which impacts the parameters of the prediction model.
To make things worse, a large part of the population may be infected without showing any symptoms. When passengers of the quarantined Diamond Princess cruise ship were tested, disturbing picture. 52% of the persons tested positive for COVID19 (N = 326) on the ship did not have any symptoms [2]. So the actual number of infected people may be vastly underestimated, making the case for social distancing even more prudent. To counter these problems various testing strategies have been devised, but the problem remains. Making accurate forecasts based on such incomplete data is problematic, to say the least.
Now what?
I think it’s clear why it requires years of study and a Master’s degree before one can start to make some sensible claims on the spread of this virus. So should we abandon making data driven models in the face of these high levels of uncertainty? No, making sense of the data remains a valuable activity, when done with expertise and adequate data. Even though accuracy and reliable forecasts are difficult, predictions provide decision-makers with a framework for action. What happens if we do nothing? Or if we lockdown? The accuracy of the models is less relevant, the general direction even more so.
Using simulations of government interventions epidemiologists distilled the now “famous” flatten the curve strategy. You can download the code to see for yourself how this strategy came about [3]. That is where the real value lies in doing these analyses, it helps decision-makers value different options under high levels of uncertainty.
Thank you
As our prime minister put it so acutely “I need to make 100% of the decision with 50% of the information”. Let’s take a moment to appreciate the difficult task our statisticians, epidemiologists and scientists have. Making sense of this complex situation with incomplete, messy data with highly complex models is hard. The implication of policy and high levels of uncertainty is a hefty combination that must weigh heavily on the shoulders of our scientists. I for one, am very thankful we have a well-trained, smart team of people equipped for this difficult task. So to all epidemiologists, statisticians, and scientists: THANK YOU!
Footnotes
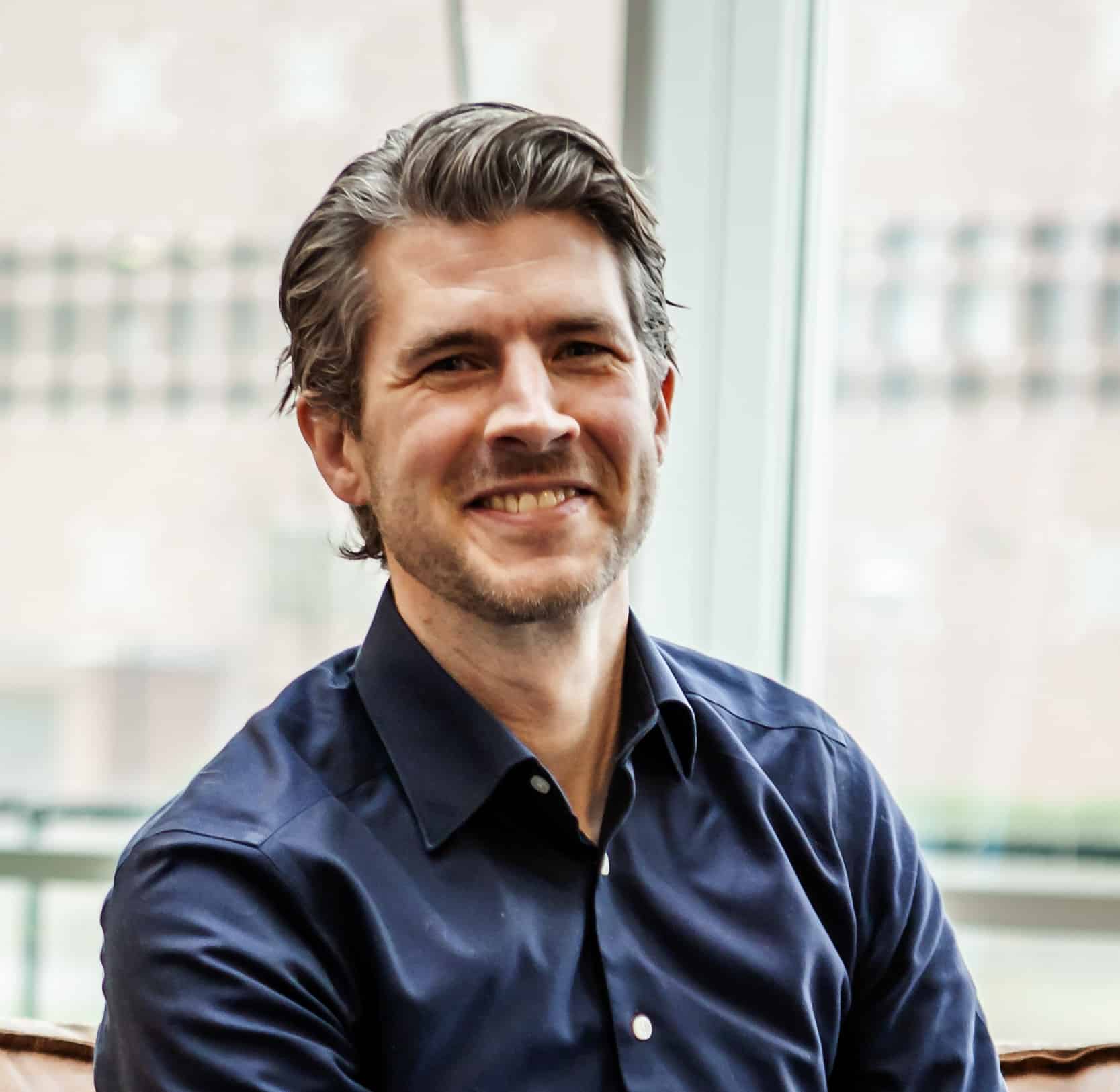
Bob Rietveld
Head of Growth Analytics
Related posts
Strategies to counter disruption or to become a disrupter yourself
We see the same things happening in the business world.…
January 7, 2021
Early warning signals for disruption
Any industry can be disrupted. Netflix is a famous example.…
December 15, 2020
The Great Reset: Disrupt Or Be Disrupted
We live in times of unprecedented change. In order to cope…
December 3, 2020