Over the course of the last weeks we, at RevelX, have been involved in helping a local hospital predict how much IC capacity is required at what point in time. Not our usual cup of tea. Normally our work involves predictions of customer lifetime value (CLV) and other more customer-oriented analyses. So on the surface, these worlds could not be further apart. But, a closer look reveals that some patterns and methods are not so different, two examples to illustrate this point.
First, Everett Rogers in the early 60’s described the pattern of how innovation spread, giving us the now-famous diffusion of innovations model. The yellow line (cumulative) describes how a product’s market share evolves over time. Now have a look at the spread of Covid19 in China, it follows a rather similar pattern. While vastly different both phenomena are based on the principle of human interaction. With innovation, there is an imitation effect where an innovator “infects” later adopters and the innovation “spreads” through the population.
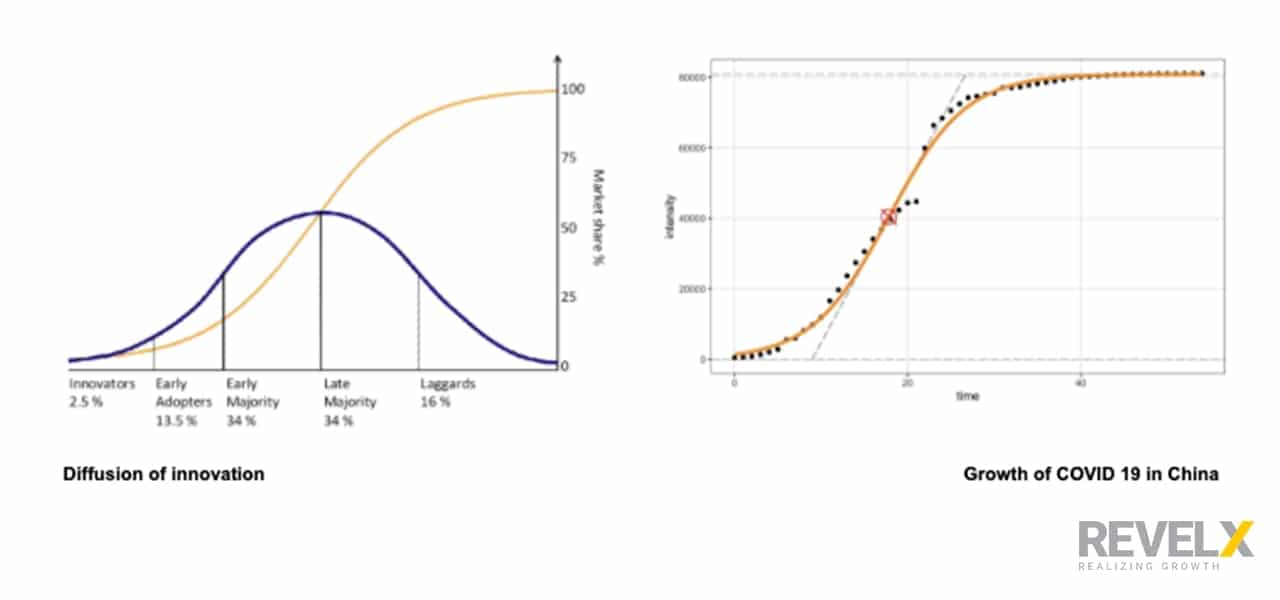
Second, take customer churn, predicting when a client will leave the company can be done using survival analysis. Churn rate is an integral part of determining customer lifetime value. Originally developed for clinical trials in the medical discipline, survival analysis models the expected duration of time for an event (churn, death, etc) to happen. As with most methods in the marketing discipline, a lot is borrowed. So epidemiology and marketing may sometimes be more related than meets the eye, although both disciplines are different domains and should be treated as such, still one can learn from crossing disciplines to see how problems are addressed.
Decisions under uncertainty
Apart from the models, COVID19 and customer lifetime value both involve making decisions under high uncertainty and changing circumstances. To ease the uncertainty and provide a framework for action simulation models can help. The flatten the curve strategy is a result of such a simulation study (Anderson et al. 2020). Just as the simulations of COVID19 provide a framework for action, so could customer lifetime value analysis for the future state of a business. As customers shift their budget due to COVID19 different questions become immediately relevant. Can you identify customers who are likely to churn and design a win-back strategy? Should you now actually spend your marketing budget on premium clients to keep them onboard for Q2/Q3?
Using up to date customer transaction data, CLV analysis can provide insights and help create a framework for action. If you need a hand running different scenarios and creating some clarity amidst this market turbulence, please let us know. It would be nice to focus on CLV once more and leave the whole COVID19 behind us.
If you want to know more about CLV of customer-based analytics, let me know at bob.rietveld@revelx.nl or in the comments.
Further Reading
If you want to know more about CLV models I can recommend the book “Customer centricity playbook” by the godfather of CLV prof. Peter Fader.
For a more academic view please see my curated selection of papers here: https://paperpile.com/shared/Z0Vi5Q.
References
Anderson, Roy M., Hans Heesterbeek, Don Klinkenberg, and T. Déirdre Hollingsworth (2020), “How Will Country-Based Mitigation Measures Influence the Course of the COVID-19 Epidemic?,” The Lancet, 395, 10228, 931–34, doi:10.1016/S0140-6736(20)30567-5.
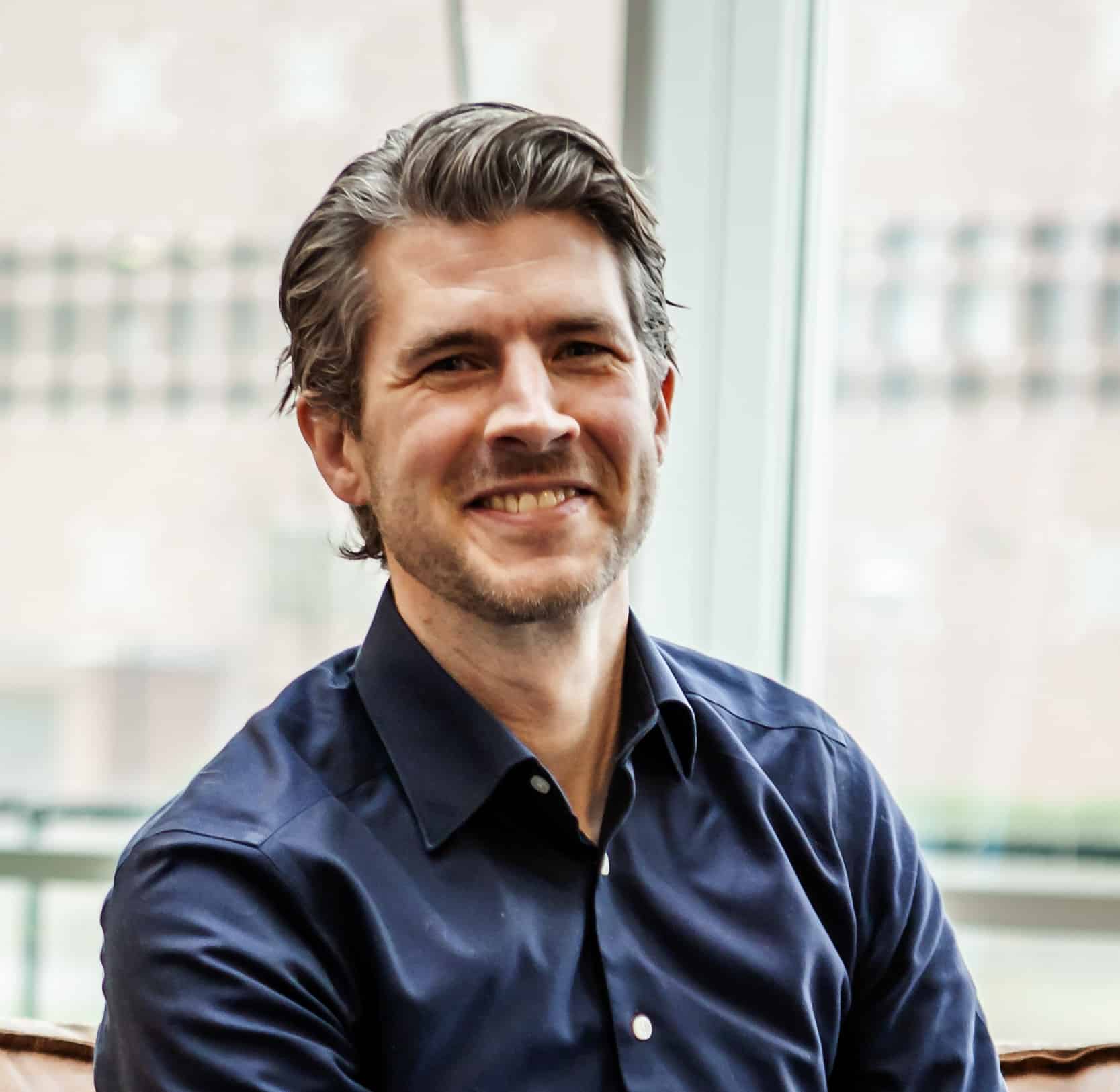
Bob Rietveld
Head of Growth Analytics
Related posts
5 Growth Mindset Examples That You Can Use in Your Job Tomorrow
People who believe their success is based on hard work,…
September 15, 2022
Retention strategy: Keeping the right customers
Retaining customers is a top-line priority for any…
May 15, 2020
Who are my most valuable customers?
Legend has it Alfredo Pareto noticed something unusual in…
April 22, 2020